Data analytics is a way to help us take advantage of technologies in ways we haven’t been able to in the past. However, oftentimes, we get the question: what exactly are data analytics and how do they apply to our digital transformation? That’s what we want to talk about here today.
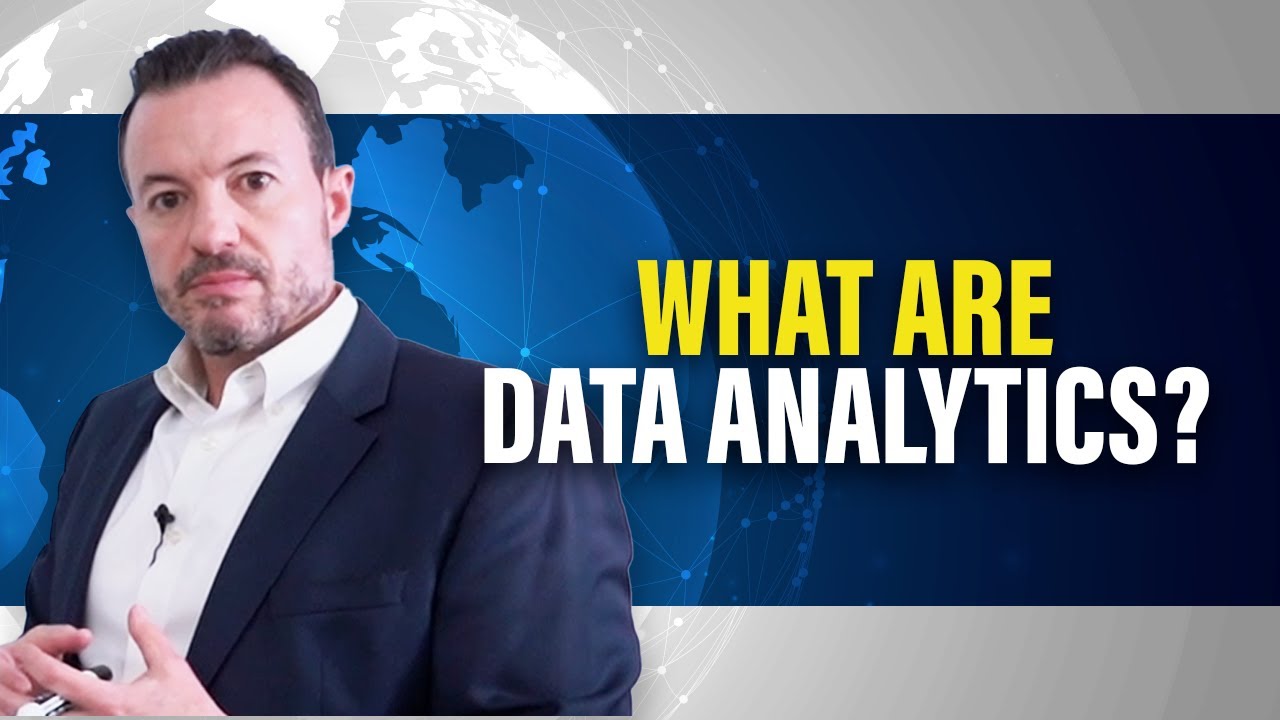
One of the common areas that we look at and evaluate when helping our clients through their digital transformation is data analytics. Data analytics is becoming more and more important as organizations grow and accumulate data over decades of using enterprise technologies. When you combine that with the fact that data toolsets and data itself are proliferating at a breakneck speed, you can see how data analytics is becoming increasingly important. In other words, organizations have more data than ever before, they’re accumulating data at a more rapid pace than ever before, and data analytics is a way to really make use of that commodity and asset that organizations are developing.
So, what I want to do today is whiteboard an example of a sample organization and talk through data analytics in the context of that organization.
Table of Contents
ToggleSample Organization
In order to fully unpack and understand what data analytics are and how they apply to an organization, it first helps to paint a picture of what a typical organization looks like. Just a real simple flow of information. So, to start, most organizations will typically have their customer. This is typically the first piece of the equation – the customer or stakeholder that they’re trying to address or provide a product or service to. Let’s just assume that this is a manufacturing organization. Even if you’re not a manufacturing organization, it’ll still help you to understand what data analytics are and how they apply to organizations. But to start, let’s assume that you have customer orders or customer inquiries that come into your sales group. So, sales is the first major function that would touch the customer or potential customer. A sales rep takes an order, and then whatever product is being sold now needs to be manufactured. So, we’ve got the manufacturing process here. And then, once it’s manufactured, we’re going to distribute that product or those products. Oops, it’s just one distribution. And then, distribution ultimately gets the product to the end customers. So, it’s sort of a circular loop here. You’ve got the distribution that comes back to the customer, customer places future orders, and on and on we go.
Now, within the manufacturing piece of it, you actually have a couple of little sub-processors here that are worth noting. You’ve got raw materials that typically come into the manufacturing process. And so, that entails buying raw materials from your suppliers. And then, you’ve got finished products here – your finished goods or components or whatever it is you’re producing for your customers. I’m going to take this and unpack this and talk about how data analytics can apply to this sort of situation or this sort of environment. And again, even if you’re not a manufacturing distribution organization, the idea is that data analytics can apply to any organization.
Big Data
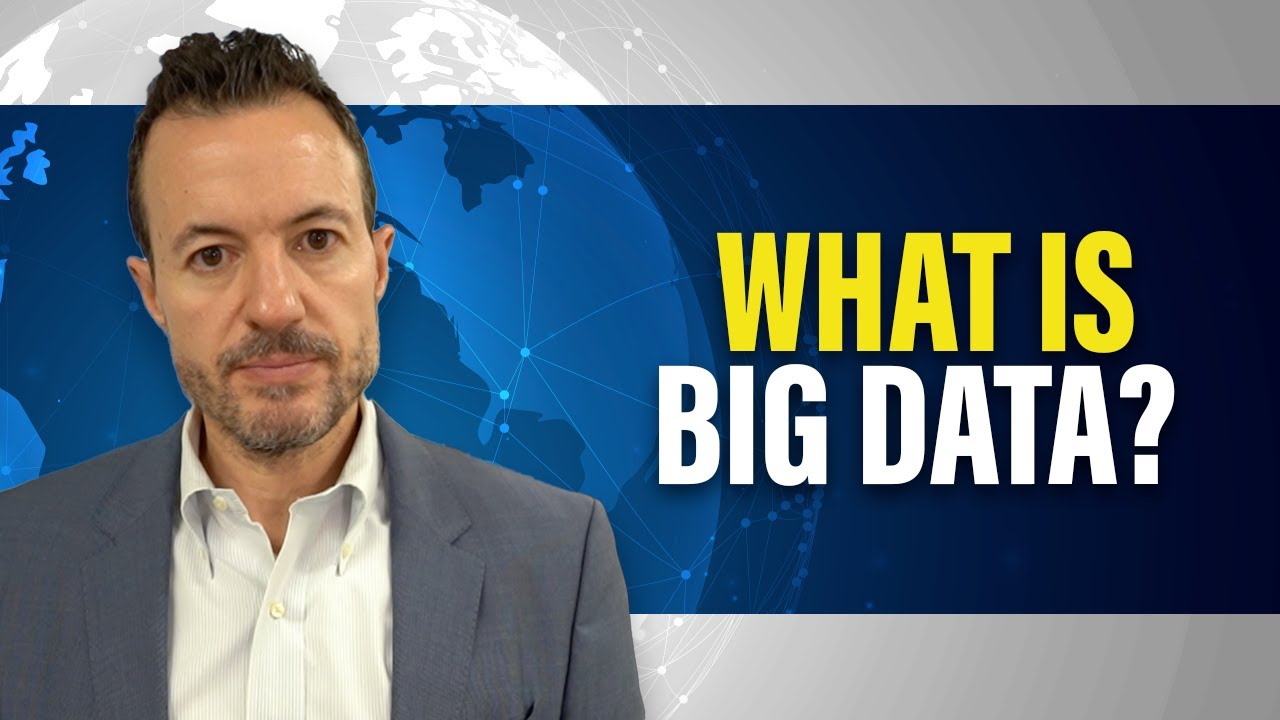
When we talk about data analytics, it helps to first understand the foundation of what goes into it. The root and source of all data analytics is big data or the data itself. Big data essentially refers to massive amounts of data accumulated throughout an organization, including the supply chain and customers. Customers generate data through inquiries, website visits, form fillings, email communication, and other interactions. Throughout the sales, manufacturing, and distribution processes, a considerable amount of data is also generated. Finance and accounting processes, which accumulate data from the supply chain, are another source of data.
Data is accumulated throughout the process, from customers all the way through manufacturing, closing the books, and accounting for all transactions that have occurred. Ultimately, the information or products going back to the customer will also produce data. Big data is vital since it captures transactional and product data, including every raw material and finished product produced by an organization, as well as every customer that becomes one.
Big data is the source and impetus for how data analytics works. Therefore, we can now talk through data analytics sequentially, starting from the most basic aspects and working our way up to the more advanced and sophisticated ones. The first and most fundamental component of data analytics is financial reporting. The Finance and Accounting group captures data to close out periods, understand what happened in terms of profitability, assign costs to appropriate accounts, and budget. The reporting includes production reports that show how many or what percentage of orders were fulfilled on time, the average lead time for customers, the average cycle time, and other insights into what has already happened within the organization.
The limitation of traditional reporting is that it looks backward, focusing on what already happened. Data analytics, on the other hand, enables us to use the same data to look forward into the future. It requires a different approach, mindset, and technology. While financial reporting and basic reports are essential, there are other things that can be done from a data analytics perspective, as we will discuss throughout the rest of this video.
Predictive Analytics
So, taking reporting one step further is going to be predictive analytics. Predictive analytics is just what it sounds like – it’s taking data from the past and trying to predict what will happen in the future based on historical trends. For example, if a manufacturing organization manufactures swimming pools and related pool supply products, it’s safe to assume that the hotter it is within certain parts of the world, the more pools and pool products that are going to be sold. Predictive analytics would look back historically to examine trends and correlations between weather patterns and sales within certain parts of the world.
That’s what predictive analytics does. It takes historic data but then models it for the future based on different assumptions. It’s a great way to not only look backward but also to plan for the future. Predictive analytics allows us to build assumptions and estimate, for example, how much we need to manufacture. If we know it’s going to be a really hot summer in London this summer, then we know that in London or in Europe, we’re going to have to produce more pools. Predictive analytics will allow us to figure out how much we need to manufacture based on the assumptions that it’s going to be warmer in London this summer, and we need to manufacture well in advance. We need to understand that before it actually gets hotter in London, if possible. That may not be the best example simply because we can’t predict weather patterns necessarily three to six months out or however long we need for this example, but it gives you a sense of how we can use predictive analytics to plan for the future.
Predictive analytics also allows us to understand what customer demand might be, which then ultimately feeds into manufacturing. But it also allows us to figure out what our staffing might be and how we might scale our organization based on projected growth, among other things. Predictive analytics is a powerful tool that has really become popular over the last 10 or 20 years in the digital transformation space. Yet, organizations still haven’t even figured out how to do basic reporting, so they certainly haven’t figured out how to do predictive analytics as well as they could. This is an area where a lot of organizations could benefit by building better predictive analytics within their organization and their digital transformation.
Taking Predictive Analytics one step further is artificial intelligence, and artificial intelligence is becoming the Holy Grail of data analytics. This is really about making the most possible use of the data you have, not only internally within your organization but also externally by looking at your customers and suppliers. If you are a manufacturing organization with raw material suppliers, capturing data from those suppliers can help you learn, identify patterns, and estimate and plan based on the massive amounts of data points throughout your supply chain.
Another thing AI allows us to do, taking big data one step further, is to use external third-party data points to augment the data we have collected within our organization. This can be used to estimate and plan what we think will happen in the future. For example, a pool manufacturer can use data related to weather patterns, based on historic trends and where scientists see the climate in London heading in the next six to 12 months, to model demand for swimming pools in London this summer. By combining this third-party data with all the data we have internally, we can use artificial intelligence to make sense of it and plan and scale for future growth in ways we haven’t been able to in the past.
Without artificial intelligence and Predictive Analytics, in the past, we would have had to rely on instinct and travel knowledge to figure out what we think is going to happen in the future. There will always be a certain amount of that required to run a business, but with powerful tools like artificial intelligence, we can take all these masses of data that exist within our organization and throughout the world with third parties to use artificial intelligence to its full capability.
Data Privacy and Cyber Security
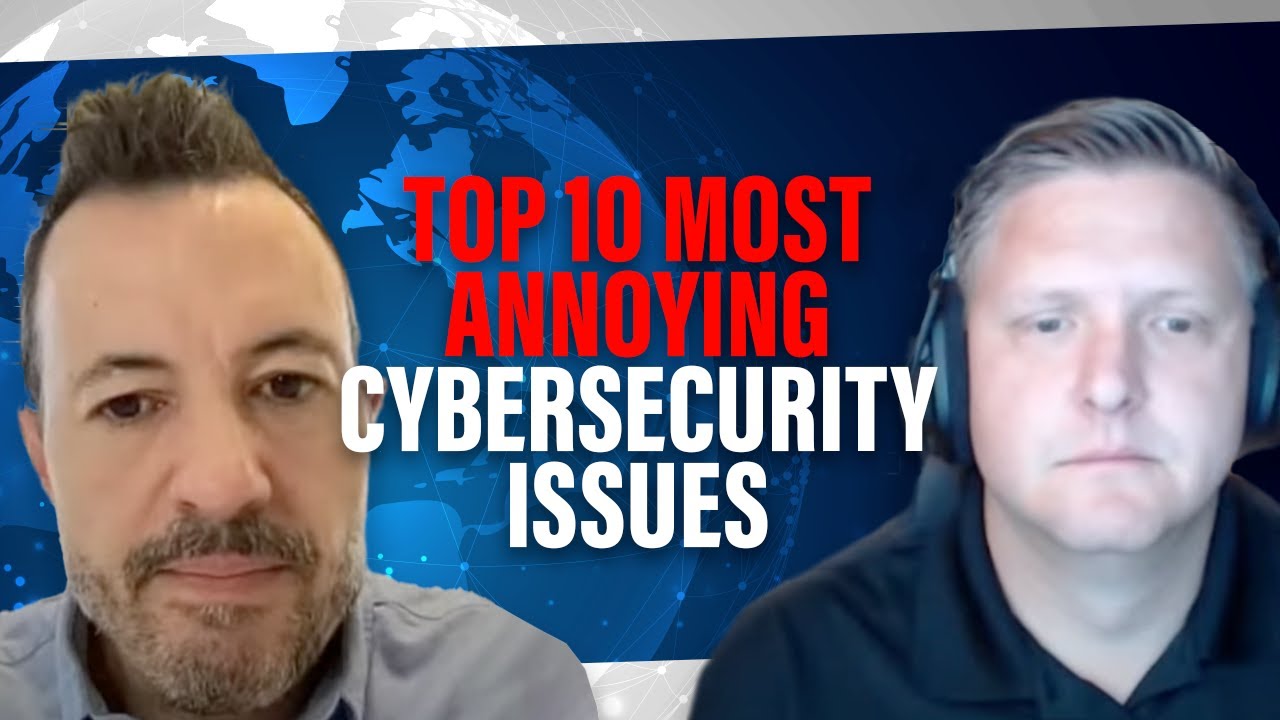
This is all exciting stuff – Predictive Analytics and Artificial Intelligence – all the stuff we’ve been talking about here today. However, there’s one risk to think about as it relates to data, and that is cybersecurity and data privacy. With masses of data and more data sources, as well as increased access to data, comes increased cybersecurity risk and data privacy issues.
Let’s start with data privacy issues. When we’re capturing information about or from our customers, there are laws and regulations that now protect customer data and limit our ability to use that data freely. For example, in Europe, there’s a law called GDPR, which limits the way we can use customer data. It’s meant to protect consumers and customers from having their data and privacy taken from them. Cybersecurity is also a concern as we look at an extended supply chain that’s much more complex than I’ve illustrated here. There are a lot of sub-steps, processes, and systems that are touched along the way. The proliferation of systems and data increases the exposure to cybersecurity threats, both internally from the organization, in terms of employees that might miss appropriate information unintentionally or intentionally, as well as externally with hackers and nefarious actors trying to steal data or do something nefarious with that data.
As we think about data analytics, we also have to be aware of the dark side, which is the data privacy and cybersecurity side of things. I hope this has given you a basic introduction to what data analytics are, how it might apply to your digital transformation.
If you are looking to strategize an upcoming transformation or are looking at selecting an ERP system, we would love to give you some insights. Please contact me for more information eric.kimberling@thirdstage-consulting.com